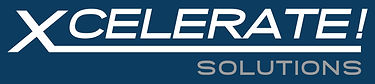
I prefer red, you prefer blue cont....
Most of your web structure & design decisions are far more complex than a simple colour choice and have the potential to impact significantly website conversion, so it is not surprising that when new ideas are broached the team will reach for that wonderful concept of the A/B test! Simple, isn’t it? Test both options and see what comes up best?
When you are looking at a simple choice, where an immediate decision is made this can be an excellent way of judging your options, however mix this with delayed decisions, returning visits, extended decision periods and suddenly the simple A/B choice isn’t so simple after all. Understanding clearly the challenges that exist in making an A/B test work effectively are vital to getting accurate results, and this is particularly pertinent for the travel sector.
In many test scenarios your conversion goal may be simply getting the visitor to click and move forward and this could be a simple decision that is taken within one session or at least over a short period of time such as 1 or 2 days. In travel, we are challenged with the situation that potential customers, when we are measuring tests by bookings, do research and take decisions over an extended period of time – 4-6 weeks being the average. Adding to this complication, we know that visitors return to an online portal multiple times to extend their research and check options. So how do you get an A/B test to work effectively?
Sample Size
Generally online travel retail experiences low website conversion rates especially when benchmarked against other non-travel retail businesses. Typical online travel companies will operate website portals that achieve 1% conversion or less. Working with a base conversion rate of 1% or lower increases the importance of understanding the sample size required to give you a statistically valid result when running A/B tests.
If your base conversion rate is 1% and you would like to detect a small improvement in conversion of, for example, 3% - you would need 2.6 million visitors to ensure that your results were significant and double if you plan to split traffic 50/50. Suddenly, your quick A/B test looks more challenging when you need to get 5 million visitors through the site to get a statistically significant result.
Outliers
Whatever test you are running and irrespective of how results are measured it is important to consider outliers and their impact. Whether your results are measured in terms of value, duration, party size etc. you need to consider the impact of outliers on either your control or test group. Imagine your average booking value is typically £1000 and suddenly you get a booking of £15000 in your test group – this one random booking could incorrectly change your view of the A/B test outcome. Smoothing the extremes of outliers is an extremely important consideration in order to maintain credibility in the outcome of your tests. It is therefore good practice to implement a method of handling these situations. You could consider removing the specific action or booking completely from your test but this has the disadvantage that it reduces what could already be scarce results either for control or test. Alternatively, you could cap the result, so in the case of our example of a £15000 booking where £1000 bookings are the norm we could count the booking in the test results but cap booking values at £3000, thereby crediting a large booking to test but without skewing results by the full value.
Control groups
It is critical that once in the test group a visitor remains there and vice versa for the control. This is very manageable when conversion goals are achieved within single sessions or even when the decision period is over a short period of time. However, in leisure travel where we are making dreams and inspiring visitors over a longer decision period with multiple visits being the norm, this poses challenges.
In order to run a valid A/B test, where you can trust the results you need to carefully consider a visitor’s entry point to your site making sure that visitors are split correctly irrespective of their entry point and making sure those splitting rules are valid.
-
Should you treat visitors that come through from meta search the same as organic search visitors?
-
What about specific landing page entrants?
-
Does a specific entry point give the test the opportunity to influence the visitor either way? How will you treat returning visitors and can you be sure they remain within their original group?
-
Can you be sure that certain web features throughout the site won’t bounce the visitor into a different group?
-
Are there visitor segments that should be removed from the test?
A/B tests can be expensive in terms of resources and time, so it is essential for you to consider all potential issues to get this right from the start – no point in realising half way through your A/B test that the participants have been mixed up.
Mobile vs web
Another major consideration for your A/B testing will be mobile especially if mobile is strategic for you and you are without cross device tracking. With extensive data protection legislation it is extremely difficult to achieve cross device tracking without breach of legislation but of course that does result in some impact to the validity of your A/B test as mobile users can flip between test and control groups as they move from mobile to desktop, a common occurrence in leisure travel at the final bookings stages. You need to consider the impact of this on the validity of your test, perhaps removing last minute bookers from the sample results to minimise impact and considering whether mobile users should be in or out of the A/B test.
Length of test
If a decision process occurs over an extended period of time, which is the nature of travel then this will complicate your test. Imagine that your customer’s standard decision period is 5 weeks for your product, then if you plan to run your A/B test over 3 months any new participant that arrives on your site for the first time after week 7 is unlikely to be counted. This means you potentially lose one third of your test participants which results in you needing to be certain that you have sufficient traffic to derive results with significance. Consider also that if you’re A/B test is major change to your site then, customers may get increasingly comfortable with it over a number of visits, so results could improve overtime and you may not see that from visitors that arrive for the first time at the latter stages of your test.
Getting a good result.
With these complications how can you navigate your way to a successful A/B test?
Starting with traffic, ensure you have enough traffic in the first place and plan to run the test over a sufficient period to get significant results. Recognising the potential problems will allow you to put measures in place to mitigate them.
-
Measure multiple data points so you can check other indicators to back up your test results eg CTR, pageviews, time spent
-
Try to define a conversion funnel so you can track progress and results down the funnel. At the top of the funnel eg booking journey you will usually have significantly more participants than at the final stages.
-
Cap the value of outliers to smooth out random disproportionate results.
-
Consider the impact of multi-device users and how to eliminate/minimize the effect – maybe removing instant bookers from the test as these will likely be users that searched on one device, but moved to a different device for the final conversion goal such as a booking.